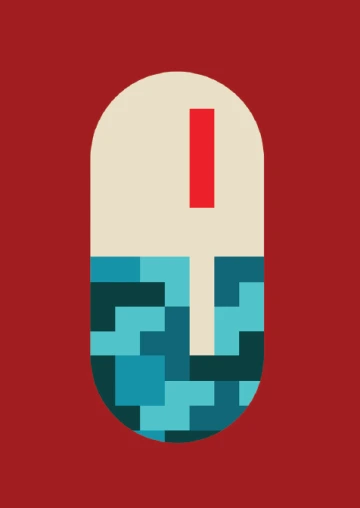
Pharmaceutical makers have historically used a highcost, hands-on discovery process – one that makes return on investment likely only for conditions that affect millions of people. As such, they’ve invested little in tackling rare diseases and conditions that mainly affect impoverished populations.
For example, the World Health Organization lists Chagas disease and dengue among 20 Neglected Tropical Diseases, so named for being “almost absent from the global health agenda.”
Now University of Arizona researchers, led by Travis Wheeler, associate professor at the Coit College of Pharmacy, are bringing machine learning to drug development to not only accelerate discovery – rapid solutions for the next pandemic, for example – but also slash costs, making research on neglected diseases more financially viable.
At the heart of this game-changing approach is a simple reality: every living cell creates and relies on proteins, which are chains of molecules assembled in ways that give each class of proteins a unique shape.
Drug molecules also have signature shapes and can only affect proteins they fit, like blocks in the game of Tetris. For example, one drug for cystic fibrosis works by linking to a key protein in a way that prevents it from misfolding out of its innate, healthy state.
Researchers are applying machine learning to hundreds of thousands of these configurations and building algorithms that swiftly scan billions of ways that drug molecules could fit to proteins in human biology.
The tools enable scientists to study vastly larger pools of potential drug molecules, making discovery faster and cheaper and increasing the likelihood of spotting a “needle in the haystack” molecule that might otherwise be overlooked.