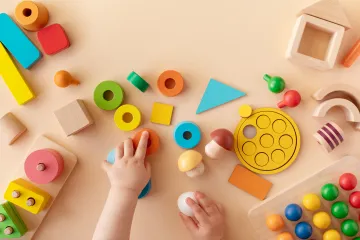
An interdisciplinary team of researchers is developing ways for non-expert clinicians to identify children at risk for autism spectrum disorder (ASD), which affects 1 in 54 children in the U.S.
Led by Gondy Leroy, professor at the University of Arizona Eller College of Management, the team combines expertise in information science, pediatric medicine and public health. The researchers are using machine learning to mark the electronic health records of children at high risk for autism based on observed behaviors that align with a medical diagnosis of ASD.
Early diagnosis and treatment drives the best long-term outcomes for children ultimately diagnosed with ASD, a condition that today is often identified late or missed entirely in childhood.
Tools from the research, funded by a $1.5 million health information technology grant from the National Institute of Mental Health, will drive earlier referrals for expert evaluation and will be especially valuable in smaller towns and rural areas, which are home to fewer physicians, especially those with specialized training for early detection of ASD.